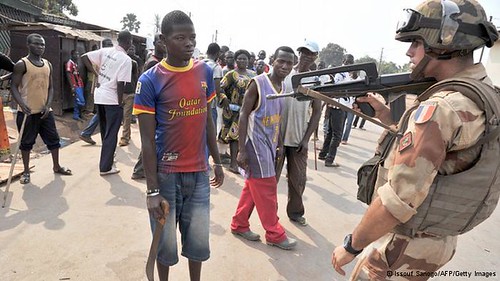
French troops holds gun against civilians in the Central African Republic. The country may be occupied by another contingent of EU soldiers., a photo by Pan-African News Wire File Photos on Flickr.
Political Scientist Forecasted the Central African Republic Genocide
Pacific Standard: The Science of Society
A conversation about the grim business of predicting mass atrocities.
After four years of intermittent peace deals between rebel groups and the government, stability in the Central African Republic (CAR) looked to be almost within reach in 2012. The qualitative evidence, at least, seemed to indicate that University of Sydney political scientist Ben Goldsmith’s Atrocity Forecasting Project modeling was deeply flawed. Using historical data from 2008 through 2010, Goldsmith and his government-funded team of computer scientists produced a 2012 analysis that ranked Central African Republic as the country with the highest risk of genocide between 2011 and 2015. Was it just a coding error?
No other prediction placed CAR so high on its rankings. One prominent model didn’t even list it. Even the Central Intelligence Agency’s preferred working group had the country positioned a good distance from the top of its fragility list.
Pacific Standard recently spoke with Goldsmith about the morbid business behind his highly accurate forecast.
How did you get interested in forecasting genocide?
One of my core areas [of research] is international conflict. I got into studying instability, internal instability within countries, and then mass atrocities or genocide, initially from an interest in the methods. And in particular, in applying various what are called machine-learning methods, that are mainly developed in computer science now. In the past, I had published one paper using the machine-learning approach and collaborating with computer scientists on international conflict. And I found it’s really quite interesting, and some of the leading political scientists who focus on statistical methods had sort of recommended machine-learning approaches, like neural networks, to study these things, instability and genocide.
I mean, for me, the biggest advantage is they’re very complex in the way they can model things in non-linear, whereas most statistical approaches sort of require you to impose linearity on the things you’re modeling. And so we got the grant [from the Australian government], and from 2010 to 2012, we worked on the forecasting project. And the forecasting aspect really also came out of what the people in the U.S. were doing with forecasting instability and forecasting genocide. So we’ve definitely built on this group, the Political Instability Task Force, and their work, and simply tried to contribute to the process. And it’s, yeah, it’s gruesome, but it’s important I think to give early warning.
You mentioned that there’s a go-to government model for this. I was looking at it earlier, and it didn’t have CAR on it. I was wondering if you could talk a little about why the models perform differently, and why yours forecasted CAR, whereas the other one didn’t.
I think the advantage of our model, or an improvement and a difference, is two things fundamentally. One is that we really tried to incorporate time-variance actors for predictors into the model more than had been done by Barbara Harff or others. And we scoured the academic literature for things that vary year in and year out, or have greater change over time, because it just seemed to me pretty clear that that could be a next step. I mean, you’re creating sort of a structural model that tells you the underlying risk, but if you’re going to forecast—which they were doing—every year, you want variables in there that vary year in and year out, so we looked at things like elections, and changes in the number of troops in the military, or changes in the economic situation—which actually didn’t prove to be very powerful—conflicts in neighboring countries, and things like assassinations of political leaders.
So one is making it much more dynamic, or time-sensitive, and another was thinking about the process in two stages. So, a lot of the recent literature on mass atrocities and genocide—and really I think it’s pretty much safe to say it’s a consensus, with the exception maybe being the Holocaust itself in World War II—but these things tend to come out of internal conflicts or deep instability and violence in a country for various reasons. So we figured we would model that directly and then include in the second stage the risk of that sort of instability as a predictor of genocide. The opposite, or the other approach, and the one that’s been taken, I think, if not universally, almost universally, is to do what’s called a conditional model. So you take only the countries that are experiencing instability, and then within that small set of countries, you try to predict which one will blow up into some sort of mass killing. And I thought that that’s really not useful, not as useful as it could be, for forecasting, because you don’t know—some countries it happens almost simultaneously, where the civil war starts and immediately they start trying to kill large numbers of a whole group based on their ethnicity or their political identity. So that happened, for example, in Cambodia in the killing fields. You know, Pol Pot knew what he wanted to do, and the civil war was all part of the same plan, basically. So you can’t forecast that if you have a conditional model.
In terms of the definition, what were you working off of? Is there a number of deaths that needs to emerge or is it something else? What exactly counts?
That really does get at one of the issues. So the definition that exists is one developed by Barbara Harff and another scholar named Ted Robert Gurr. They first published it I think in the ’80s. And it doesn’t specify a number of deaths, it specifies targeting a particular group. And it’s just based on the idea of targeting the group because of who they are. And therein sort of lies the difficulty of well … if you target three people, is that really the same as Cambodian genocide?
So people like Ben Valentino, who’s at Dartmouth, are arguing that really what’s more … what’s a better thing to study is mass killing. When there’s mass killing of civilians that reaches above a certain threshold, so a thousand people or 10,000 people, then that’s something that can be more reliably coded and we can study and forecast in a systematic way. I actually tend to disagree with that. I think that mass killing is too broad a category, and what’s distinct about some things is that, some of these events, is that they target particular groups just as you would expect from the definition of genocide, let’s say you find in the international law, which is not a very useful definition for social science, but targeting a group in whole or in part with the idea of eliminating those people for some political end.
So we use the dataset that’s been developed based on this definition, but there’s certainly room for improvement in the coding. But I don’t think just having a threshold—because say, the Russians fighting in Chechnya, they carpet-bombed the Capital city, Grozny, and killed thousands and thousands of civilians, but most of them were ethnic Russians. And it’s not the same sort of thing, and I think it doesn’t have the same causes and predictors.
Why do you think, in particular, CAR came up so high on your model versus something like Syria?
One thing, because the models are so complex, it’s a bit hard to answer that question beyond looking at the values of the different factors. But CAR stands out because they did have changes in the peacekeeping troops. So they had peacekeepers there that were, I forget the exact sequence now, but brought in and then withdrawn, or vice versa. They had an election—so this is looking at the years 2008, 2009, 2010, that are the last years of the data before we produced our forecast for 2011 onward—elections scheduled, which were then, I believe, canceled. And they were very high on some of the more structural variables as well, like the ethnic fractionalization and the infant mortality. So that combination of risk factors clearly puts it up at the top of the model.
And that’s sort of as much as we can get. One caution about the models is that they’re really designed for forecasting and not for interpretation. Because the operationalizations, you know, the way the variables are coded and the way they interact, is very complex. And so it’s actually quite difficult to say, “Well, this went up and therefore it increased the risk.” It could be one factor in combination with another, and we’ve optimized the model for forecasting on historical data, but in that process, we’re not doing what you would do in proper social science: a well-specified model based in theory that focuses on a particular variable and tries to talk about causation and causal mechanisms. So, that’s sort of a different sort of work.
It’s almost a funny curiosity that you want your model to do well, but you also maybe don’t want it to do well.
It’s an odd situation to be in. Well, I mean there are two things. One, of course, you don’t want these things to happen. And you look at cases that seem unlikely and, of course, you think, you hope they are actually unlikely. And Myanmar was another. I really thought we just missed it because we didn’t get enough of the liberalization process in our data. But actually, it’s that liberalization that seems to perhaps make it more at risk. And we had the regime change in the last few years as one of our time-sensitive variables. So it’s that—wanting the model to do well, but really not.
But then there’s also the sort of very difficult issue from a point of view of assessing the accuracy. With the rise of the norm of responsibility to protect, it seems to have some effect that states—the U.S. and European states—actually want to step in and prevent these things. So, if the states intervene, when our model says something is dangerous, does that mean our model fails? Of course, we want them to intervene, but then, you can’t assess the accuracy of the model.
Is there anything that, as you’re working on these models in the future, that you’d like to see happen?
So there’s a project that used to be at Harvard but is now, I think, based [somewhere else] called the Satellite Sentinel Project. I think George Clooney is one of their funders. And they do monitoring, in particular, in Sudan, and Darfur was one of their important cases. And they use satellite images. And so that’s sort of intensive monitoring, and they’ve developed a whole methodology to really track in real-time what’s going on. I visited them, and it’s really quite chilling. They can show you how the trucks show up, they surround the village, then the next day there are body bags stacked up. It’s really, really horrid to see. That sort of monitoring is not something you can do for every country that might be at risk based on just a qualitative assessment. But with a shortlist, like those that we produce or others produce, you can focus. The U.S. government can afford to do that for 10 or 15 countries. So it’s that sort of monitoring and then the awareness of the high risk that I think can really make a difference.
No comments:
Post a Comment